Artificial intelligence has erupted across markets, promising organizations the capability to transform and optimize the business rapidly. Defined, artificial intelligence is the “development of computer systems able to perform tasks that normally require human intelligence.” Although techniques such as machine learning (ML) and deep learning (DL) are increasingly utilized within the utilities sphere, AI in the sense of true, autonomous artificial intelligence is still immature— and the areas of ML and DL are still experimental in many cases.
Yet, significant opportunities do persist across the utility value chain today. Use cases in customer operations, smart grid, outage management, asset management, supply chain and market operations (to name a few) hold enormous potential for utilities.
Near term AI use cases
AI, particularly ML and DL, is playing a role at many utilities today, and those capabilities are posited to evolve and expand. Following is a list of example use cases where AI has transformative potential in the near term:
- Predictive Maintenance: Pattern recognition via machine learning has been widely adopted in asset and infrastructure management. AI will enable utilities to understand more of their assets in real time further and further downstream, allow them to forecast and test extensive maintenance scenarios, and ultimately optimize their operational planning around assets. Specific use cases such as predictive and condition-based maintenance (CBM) for assets take advantage of the ability to identify and correlate patterns with past events and performance of similar assets to predict asset health in real time, determine more accurate maintenance requirements, and eliminate the risk of catastrophic failures.
- Visualizing assets and vegetation: Drones and other image-gathering technologies are becoming widespread, but insufficient manpower limits the ability to analyze images and video. Artificial neural network technology can alleviate that stress by classifying assets and detecting asset flaws. It also can support vegetation management by determining the type of work that needs to be done, helping utilities to prioritize that work more effectively.
- Smart Grid: AI is an expanding area for system management and control optimization automation. Capabilities such as automated lateral switching, FLISR, and the ability to integrate and optimize DER will improve rapidly with the expansion of AI techniques. The Department of Energy recently announced a plan to leverage AI in developing an autonomous grid. One early application will incorporate data from satellite imagery, operational and geospatial systems, meters and other network devices to build a simulated model of the electric distribution system that can proactively identify areas of vulnerability and prioritize action.
- Customer experience and engagement: Like many industries today, utilities want a 360-degree view of the customer but are limited by inadequate data. AI can help build out a more refined view of the customer along many dimensions, including propensity to behave in more specified ways, under variable conditions (e.g., file complaints, sign up for programs, or not pay their bill). Propensity models can go further to inform recommendation systems. For example, a CSR can be told to make a proactive call to a customer, and/or can be informed of a “next best action” to pursue when they are already on the phone with someone (for example, program enrollment or offering rebates).
- Safety and operational reporting: Safety and other operational departments at many utilities struggle with the ability to transfer written information to usable data. As a result, valuable intelligence remains isolated and unusable. Document automation can be enabled by natural language processing to eliminate transcription and data entry efforts that might otherwise be impossible or take years to complete.
- Fraud and revenue protection: Many utilities are already heavily invested in targeting these areas through the observation of patterns and usage. In most cases today, human intervention is required to verify what the analytics have found. AI efforts are growing to minimize human intervention and enable these processes to be more autonomous.
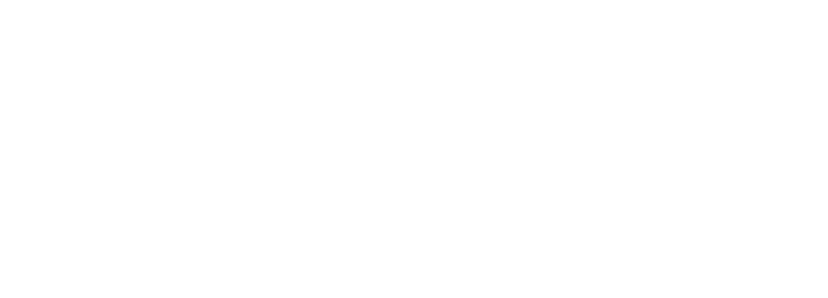